What Are Some Best Practices And Tips For Image Annotation?
As technology continues to evolve, it's no wonder that image annotation has taken significant strides. Nowadays, it plays a vital role in daily life, from simple activities such as unlocking your mobile phone to robots performing numerous tasks in various industries.
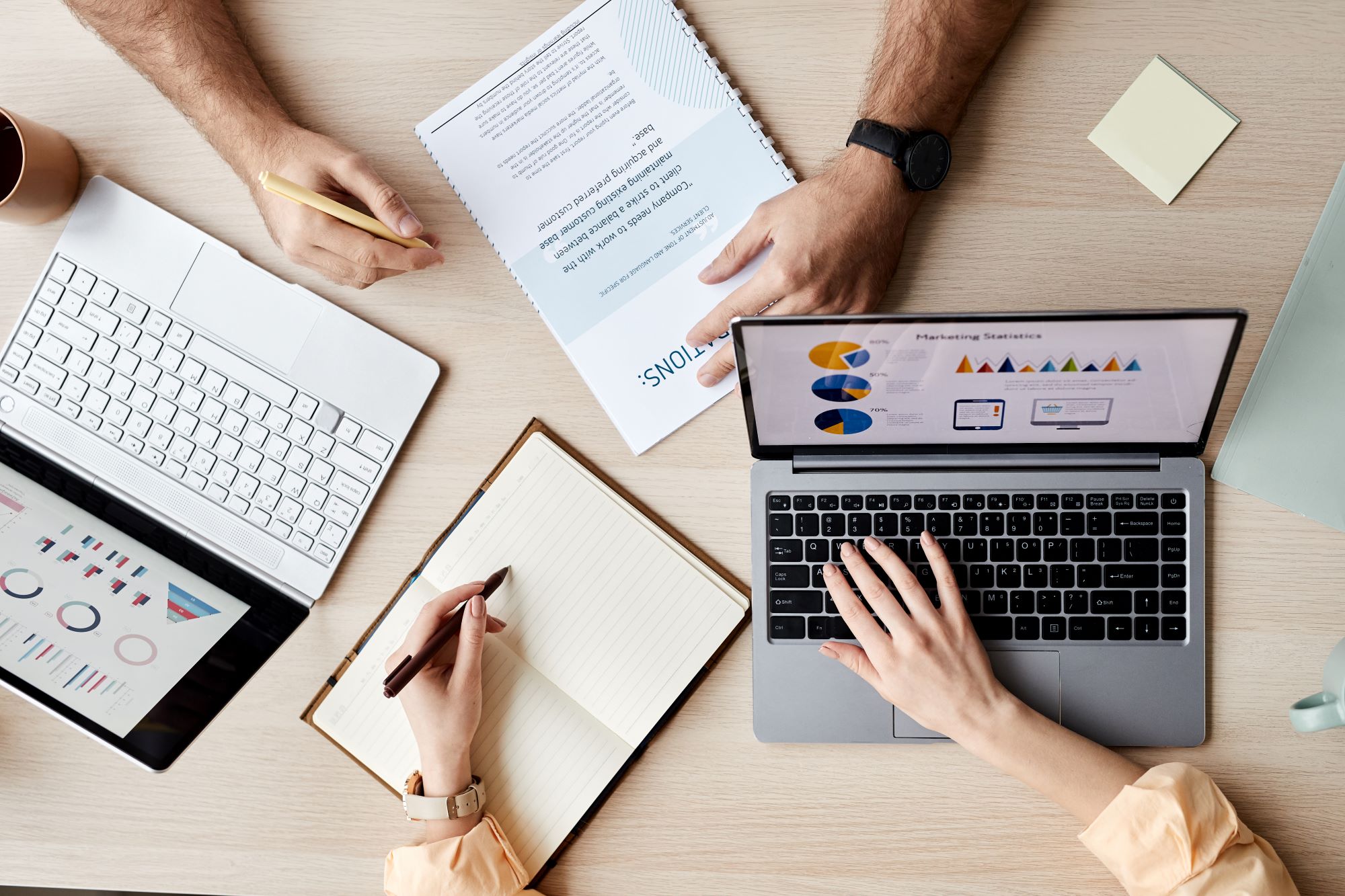
Some of the industries and applications that utilize image annotation include security, surveillance, medical imaging, drone imagery, robotics, and autonomous vehicles, to name a few.
Understanding Image Annotation
Image annotation involves appointing labels to images. As part of the process, the manual work involves assessing the set of images, pinpointing pertinent objects in every image, and annotating them. For example, when indicating the color and tag of every object, you can utilize image annotation to generate a training dataset.
The models utilize annotations to learn to recognize objects or label images independently. The process is highly valuable in training models for different undertakings such as image classification, object recognition, and image segmentation.
When it comes to the labels, the numbers will vary depending on the type and scope of the project. For example, a single label can represent an entire image. In other cases, it involves identifying specific objects. The best way to maintain accuracy with the labeling is by allowing several annotators to label the same image, with the majority voting to choose the most fitting label.
Best Practices And Tips For Image Annotation
When working with machine learning models, image annotation is an important step. A model can only correctly identify objects in videos or images with precise annotations.
Implementing best practices is an effective way to ensure your image annotation projects flow seamlessly. Here are several best practices to remember to get the most out of image annotation:
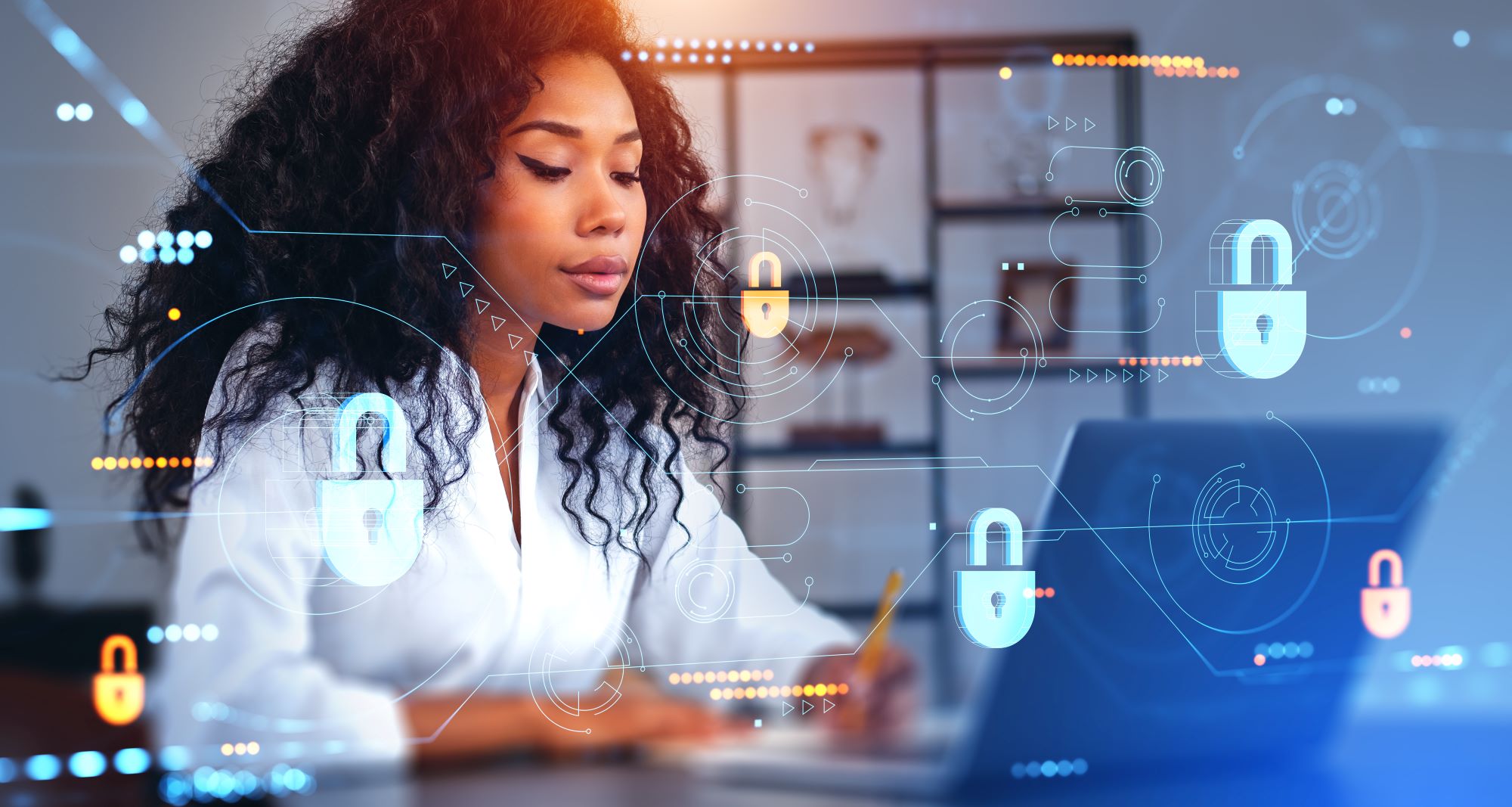
1. Create A Simple Labeling Approach
The best way to maximize image annotation is to have a strategy. Without one, you might miss out on labeling opportunities that may help boost your model's performance. A solid labeling strategy will depend on your specific objectives, available data, and the types of labels you want to appoint.
While doing it, it's crucial to ensure that image annotation projects stay uniform and straightforward. Make sure to utilize the same format and terminology for all the labels. Remember to preserve consistency when it comes to punctuation and capitalization. Decide what pattern works and implement it throughout the labeling process.
2. Employ High-Quality Data
If you want to ensure the optimal performance of your model, make sure to use top-quality data. With this in mind, consider investing in good-quality training datasets. For example, when utilizing an image labeling tool to work on a set of images, they should represent variations in what you want to label.
The creation of training data should include all possible use cases. Generally, it involves utilizing high-quality images from all angles with the right lighting conditions and distances. It's crucial to know that these factors are key to training models that are more accurate and robust. Furthermore, make it a priority to train annotators with suitable expertise and knowledge in their respective fields.
3. Assess The Data For Reliability, Diversity, And Precision
When determining the reliability and accuracy of the machine learning model, it's crucial to determine how it performs over numerous tasks. The ideal approach is to develop a trial dataset with positive and negative examples. Use your annotation tool to annotate the examples and compare how it can get them right.
4. Opt For The Appropriate Annotation Tool
The right annotation tool should be easy to use. It should allow collaboration and readily integrate into your current workflows. Remember that working with an annotation tool that seamlessly aligns with your objectives and workflow is crucial.
It may be best to utilize a bounding box annotation, depending on your project. It usually works best when handling large data volumes requiring swift labeling. Consider using a segmentation tool if the project involves specific, crucial areas to label accurately.
5. Select A Fitting Platform For Your Data Annotation
Nowadays, choosing from an array of data annotation platforms can be overwhelming, each with distinct features and limitations. When selecting one, the best way to make this task manageable is to consider your objectives, preferences, and current workflows. The right platform is the key to helping you annotate efficiently.
You need to become more familiar with the features. Learning how it functions before labeling data is crucial. Today, some platforms include tutorials and training to familiarize you with the features. Other annotation tools may require further training before you start your labeling project.
It's also best to consider using an annotation platform with machine-learning capabilities. Generally, machine learning tools can understand previous annotations and readily apply what they've learned to new ones.
6. Maximize The Use Of Active-Learning Methods
Using active learning is the best way to identify suitable examples to annotate. It's an efficient method of annotating data and can be utilized for various annotation tasks such as image classification, object detection, and segmentation.
The active learning techniques utilize a machine to determine suitable examples for annotation. Generally, it helps reduce the number of manual annotations necessary to train your model. It also cuts down on the manual action required to train data sets.
The active learning approach can significantly improve your models by annotating a subset of data likely to hold critical information.
7. Observe Accurate Labeling Or Tagging For Obscured Objects
Objects partly obscured in an image and out of view are known as occluded objects. In such cases, ensure it's appropriately labeled in the same manner as when it's available in full view.
A common mistake when there are occluded objects is drawing bounding boxes on the partly visible part of the object. The boxes may overlap if more than one object appears obscured.
Final Thoughts
Today, the applications for image annotations are endless, ranging from security to surveillance, and even robotics, to name a few. Although there are various components to image annotation, the entire process involves the production of good-quality data for model training. Knowing about the best practices will ensure the best outcome for whatever project you're currently working on.