Do You Really Need a Big Data Warehouse?
The huge data warehouse (HDW) is a key storage component of the architecture of a big data solution; it differs from the conventional DWH in the following respects:
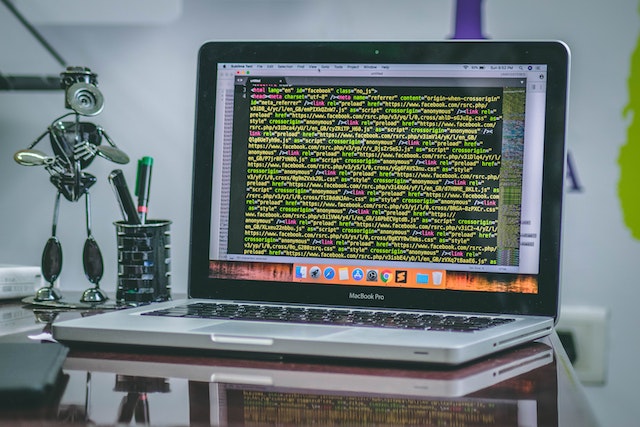
1. Form of Information
The conventional DWH only keeps identical records, such as those found in CRM, ERP, etc. The big data warehouse acts as a universal repository for a wide variety of data types, including but not limited to conventional data, heterogeneous big data (such as transactional data, sensor data, weblogs, audio, video, government statistics, and more), and more.
2. Statistics of information mass
Business-level data warehouses are limited in their ability to process massive amounts of information (typically, they store terabytes of data). Big data warehouses can store several petabytes of data, if not more. Managing data at such levels requires careful planning, and in this article, we outline the steps our clients can take with the help of the right technological stack.
3. Method for Assessing Data Quality
The conventional DWH needs reliable information that is also comprehensive, correct, and easily auditable.
When discussing the quality of large data, it is difficult to satisfy the aforementioned conditions. To get the massive data warehouse's data to a 'good enough' condition, professional data analyst consulting firm establish minimum suitable standards. The values of these thresholds change from one activity to another. Consider the case of huge data and the need for fullness criteria. It is not necessary to have two days' worth of data in order to identify client mood throughout the fall season when evaluating buying patterns in social media. In the oil and gas industry, however, - the lowest suitable thresholds will be greater since, without the two-day quantity of data, you might miss certain key trends that can lead to equipment malfunctions or oil spillages.
4. In the Stack of Technology
Technologies such as Microsoft SQL Server, Microsoft SSIS, Oracle, Talend, Informatica, etc. are used in the conventional data warehouse.
Amazon RedShift, HBase, Apache Kafka, Apache Spark, HDFS, Hadoop MapReduce, Apache Cassandra, etc. are only some of the technologies used by the big data warehouse to handle massive amounts of data storage, near-instant streaming, and parallel processing.
5. Insights
Advanced analytical technologies based on artificial intelligence, such as machine learning, are made possible by the design of huge data warehouses. Companies may get more insight into how to improve business operations, make reliable forecasts, and come up with actionable recommendations by analyzing large amounts of data gathered from a variety of sources.
Although analytics is used in the business data warehouse, the volume of data available prevents its full implementation of the aforementioned cutting-edge technologies. It follows that all that can be gleaned from the analytics is a description of what took occurred and an explanation of why that took place.
6. Data Obtainable
While both small and large DWHs strive to do the same thing—providing insights to decision-makers—the big data warehouse goes the extra mile by making real-time information accessible to everyone in the company. In this approach, a wider range of decision-makers will have access to the insights.
Big data is where it's at
A large data warehouse is essential to any big data solution. Furthermore, a data lake may be required to supplement it. However, you can always ask DataArtt's staff for a bespoke solution if you'd rather not go into the technical specifics on the route to your big data solution that satisfies your business objectives.